Self-Consuming Generative Models with Curated Data
Best AI papers explained - A podcast by Enoch H. Kang
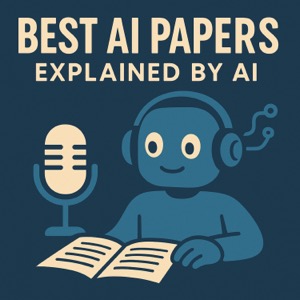
Categories:
This paper examines how curation of synthetic data, often reflecting human preferences, impacts the iterative retraining of generative models. The authors theoretically demonstrate that when generative models are trained on curated synthetic samples, the expected reward associated with the curation process increases, and its variance diminishes, leading to the model converging towards data maximizing that reward. However, this can also result in bias amplification, as shown through experiments. Stability guarantees are provided when mixing real and curated synthetic data during retraining, drawing connections to Reinforcement Learning from Human Feedback (RLHF), where the models implicitly optimize preferences. This research highlights that the increasing presence of curated synthetic data online acts as an implicit mechanism for preference optimization in future generative models.