THINKPRM: Data-Efficient Process Reward Models
Best AI papers explained - A podcast by Enoch H. Kang
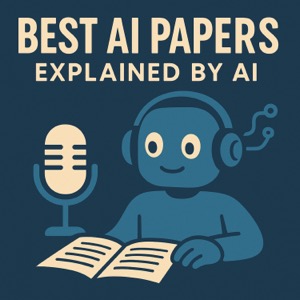
Categories:
This academic paper introduces THINKPRM, a novel type of process reward model (PRM) designed to be data-efficient. Unlike traditional discriminative PRMs requiring extensive step-by-step annotations, THINKPRM leverages the reasoning abilities of large language models by generating a verification chain-of-thought (CoT) to evaluate each step of a solution. By fine-tuning on a significantly smaller dataset of synthetic verification CoTs, THINKPRM outperforms both discriminative verifiers and LLM-as-a-Judge baselines across various benchmarks, including out-of-domain tasks. The research demonstrates that THINKPRM effectively scales test-time compute for verification, offering better performance on challenging reasoning problems while requiring minimal training supervision.keepSave to notecopy_alldocsAdd noteaudio_magic_eraserAudio OverviewmapMind Map